Apr 22, 2019 Keylogger Pack a huge collection of keylogger for all purpose Aux Logger v3.0.0.0 Monitor Hooker Perfect Keylogger PoisonLogger UltimateLogger Anonymous Keylogger DigitalKeyloggerv3.3 Dracula Logger Project Neptune v2.0 RapZo Logger v 1.5 ( Public Edition ) RinLogger Silent. ELEGOO Smart Robot Car: An educational STEM kit for beginners (kids) to get hands-on experience in programming, electronics assembling, and robotics knowledge. It is an integration solution for robotics learning and made for education. Complete Package: Contains 24 kinds of module parts including obstacle avoidance, line tracking module, infrared remote control and also you can control it via. Mar 23, 2021 Content: PoisonLogger Cain - Password Recovery Utility ARCANUS 1.5.6 Haxor - AIO Babylon RAT Anonymous Keylogger RinLogger HOIC 2.1 njRAT v0.11G Project Neptune v2.0 Digital Keyloggerv3.3 Syslogger Ardamax 3.0 Hoic 1.1 UltimageLogger Project Neptune v1.78 Hoic1.1 Celesty DraculaLogger Unknown.
If they arenât already selected, check the four checkboxes below the time delay.
Leave the âHeaderâ options as they are.For the Email Settings, I used a GMail account so my SMTP Sending Server was smtp.gmail.com with a port of 587. If you are using Windows Live, simply change the SMTP from smtp.gmail.com to smtp.live.com.
For the next box, put in your email address, then your email accounts password below that, and then your email address again in the box under that.
(NB: Do not worry, no one but you sees the email and password, but if youâre really skeptical, just spend a few minutes to make a new account to use. Remember to remember the details as this is where your victims logs go to!) To test your email click the Test Email Account Information button.
Just leave the FTP Settings area alone.
So, this is what your first tab should look like:
You can leave the âSystem Settingsâ section as it is as well. After all that, your Administrative Tab should look something like this:
Skip the last section of this Tab.
Your selections should look something like this:
As we are on the free version of Project Neptune we canât change much in the Screenshots section, but if you want to see a screenshot of what your slave is doing, check the top option. You wonât be able to affect the time between screenshots as we are using the free version.
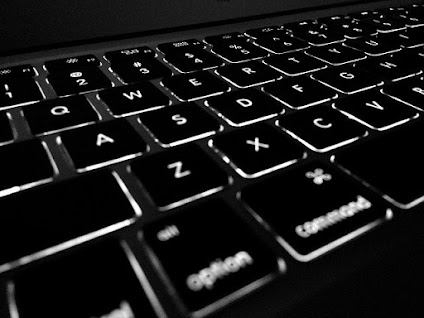
Once youâve done all that, you should have something that looks like this:
Project Neptune V2.0 Free Download Windows 10
We are gonna go skip the âApp Settingsâ tab and go straight onto the âServer Creationâ one.For the Server Settings section, you donât really need all these things but you can do it to make your .exe seem authentic. For description, put something describing what your pretending your keylogger is. I usually disguise mine as games so mine would have a description of the fake game. For company, make up some random company name.For copyright, just write something like Copyright 2012.Leave the two checkboxes unticked. Skip the File Pumping section and the Server Generation for now.
You should now have a tab that looks like this:
If there is positive feedback I may do a tutorial on how to spread your keylogger around đ
Original author(s) | Guolin Ke[1] / Microsoft Research |
---|---|
Developer(s) | Microsoft and LightGBM Contributors[2] |
Initial release | 2016; 5 years ago |
Stable release | |
Repository | github.com/microsoft/LightGBM |
Written in | C++, Python, R, C |
Operating system | Windows, macOS, Linux |
Type | Machine learning, Gradient boosting framework |
License | MIT License |
Website | lightgbm.readthedocs.io |
LightGBM, short for Light Gradient Boosting Machine, is a free and open source distributed gradient boosting framework for machine learning originally developed by Microsoft.[4][5] It is based on decision tree algorithms and used for ranking, classification and other machine learning tasks. The development focus is on performance and scalability.
Overview[edit]
Project Neptune V2.0 free. download full

Son Of Neptune Free Online Pdf
The LightGBM framework supports different algorithms including GBT, GBDT, GBRT, GBM, MART[6][7] and RF.[8] LightGBM has many of XGBoost's advantages, including sparse optimization, parallel training, multiple loss functions, regularization, bagging, and early stopping. A major difference between the two lies in the construction of trees. LightGBM does not grow a tree level-wise â row by row â as most other implementations do.[9] Instead it grows trees leaf-wise. It chooses the leaf it believes will yield the largest decrease in loss.[10] Besides, LightGBM does not use the widely-used sorted-based decision tree learning algorithm, which searches the best split point on sorted feature values,[11] as XGBoost or other implementations do. Instead, LightGBM implements a highly optimized histogram-based decision tree learning algorithm, which yields great advantages on both efficiency and memory consumption. [12] The LightGBM algorithm utilizes two novel techniques called Gradient-Based One-Side Sampling (GOSS) and Exclusive Feature Bundling (EFB) which allow the algorithm to run faster while maintaining a high level of accuracy.[13]
LightGBM works on Linux, Windows, and macOS and supports C++, Python,[14]R, and C#.[15] The source code is licensed under MIT License and available on GitHub.[16]
Gradient-Based One-Side Sampling[edit]
Gradient-Based One-Side Sampling (GOSS) is a method that leverages the fact that there is no native weight for data instance in GBDT. Since data instances with different gradients play different roles in the computation of information gain, the instances with larger gradients will contribute more to the information gain. Thus, in order to retain the accuracy of the information, GOSS keeps the instances with large gradients and randomly drops the instances with small gradients.[13]
Exclusive Feature Bundling[edit]
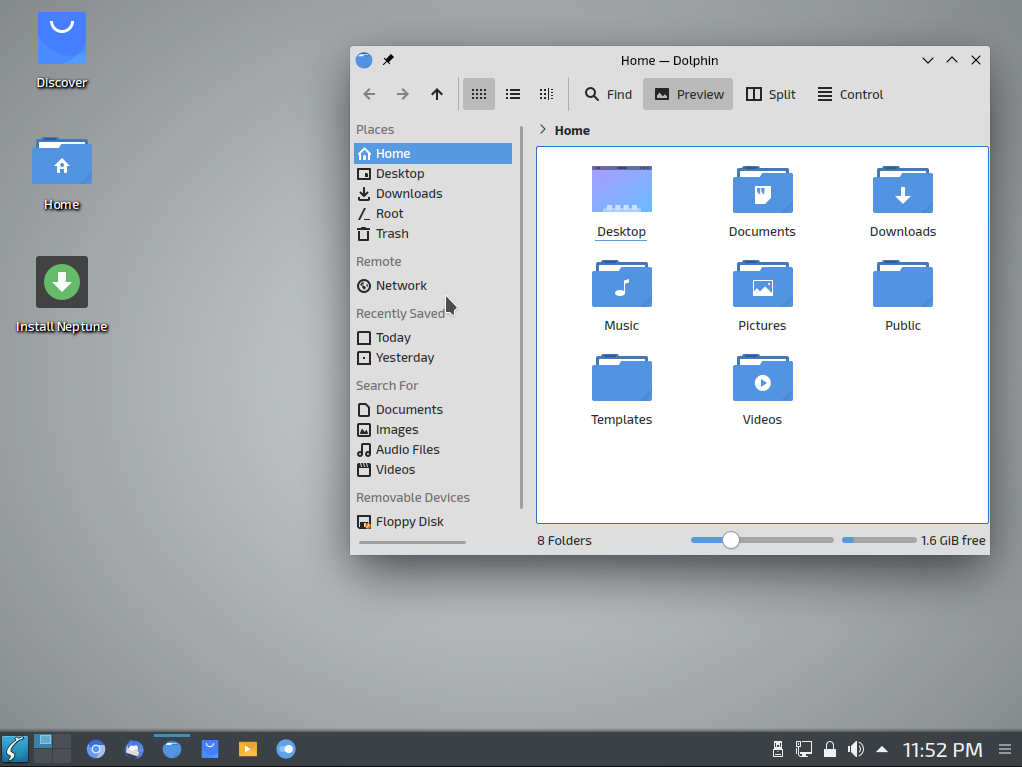
Exclusive Feature Bundling (EFB) is a near-lossless method to reduce the number of effective features. In a sparse feature space many features are nearly exclusive, implying they rarely take nonzero values simultaneously. One-hot encoded features are a perfect example of exclusive features. EFB bundles these features, reducing dimensionality to improve efficiency while maintaining a high level of accuracy. The bundle of exclusive features into a single feature is called an exclusive feature bundle. [13]
See also[edit]
References[edit]
Project Neptune V2.0 free. download full
- ^'Guolin Ke'.
- ^'microsoft/LightGBM'. GitHub.
- ^'Releases ¡ microsoft/LightGBM'. GitHub.
- ^Brownlee, Jason (March 31, 2020). 'Gradient Boosting with Scikit-Learn, XGBoost, LightGBM, and CatBoost'.
- ^Kopitar, Leon; Kocbek, Primoz; Cilar, Leona; Sheikh, Aziz; Stiglic, Gregor (July 20, 2020). 'Early detection of type 2 diabetes mellitus using machine learning-based prediction models'. Scientific Reports. 10 (1): 11981. Bibcode:2020NatSR..1011981K. doi:10.1038/s41598-020-68771-z. PMC7371679. PMID32686721 â via www.nature.com.
- ^'Understanding LightGBM Parameters (and How to Tune Them)'. neptune.ai. May 6, 2020.
- ^'An Overview of LightGBM'. avanwyk. May 16, 2018.
- ^'Parameters â LightGBM 3.0.0.99 documentation'. lightgbm.readthedocs.io.
- ^The Gradient Boosters IV: LightGBM â Deep & Shallow
- ^XGBoost, LightGBM, and Other Kaggle Competition Favorites | by Andre Ye | Sep, 2020 | Towards Data Science
- ^Manish, Mehta; Rakesh, Agrawal; Jorma, Rissanen (Nov 24, 2020). 'SLIQ: A fast scalable classifier for data mining'. International Conference on Extending Database Technology. CiteSeerX10.1.1.89.7734.
- ^'Features â LightGBM 3.1.0.99 documentation'. lightgbm.readthedocs.io.
- ^ abcKe, Guolin; Meng, Qi; Finley, Thomas; Wang, Taifeng; Chen, Wei; Ma, Weidong; Ye, Qiwei; Liu, Tie-Yan (2017). 'LightGBM: A Highly Efficient Gradient Boosting Decision Tree'. Advances in Neural Information Processing Systems. 30.
- ^'lightgbm: LightGBM Python Package' â via PyPI.
- ^'Microsoft.ML.Trainers.LightGbm Namespace'. docs.microsoft.com.
- ^'microsoft/LightGBM'. October 6, 2020 â via GitHub.
Further reading[edit]
Project Neptune V2.0 Free Download Pc
- Guolin Ke, Qi Meng, Thomas Finely, Taifeng Wang, Wei Chen, Weidong Ma, Qiwei Ye, Tie-Yan Liu (2017). 'LightGBM: A Highly Efficient Gradient Boosting Decision Tree'(PDF).Cite journal requires
|journal=
(help)CS1 maint: uses authors parameter (link) - Quinto, Butch (2020). Next-Generation Machine Learning with Spark â Covers XGBoost, LightGBM, Spark NLP, Distributed Deep Learning with Keras, and More. Apress. ISBN978-1-4842-5668-8.